New Algorithm Helps Doctors Predict Adults At Risk For PTSD: Identifying The 10% Who Find It Difficult To Heal
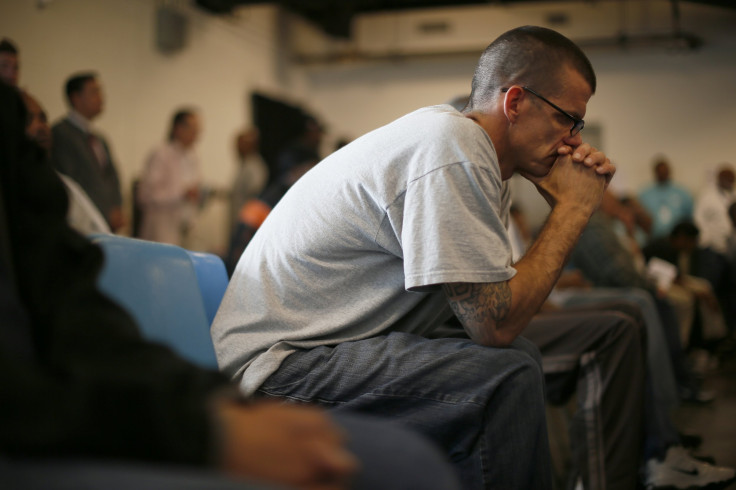
The majority of adults will experience at least one traumatic event sometime in their lifetimes, research suggests, yet only five to 10 percent will go on to develop post-traumatic stress disorder (PTSD). Doctors already know some of the factors that increase a person’s risk, yet there is no clear and accurate set of guidelines for doctors wanting to predict which of their traumatized patients will develop the mental disorder. Now, scientists at NYU Langone Medical Center have designed a new computational tool to identify people at increased risk for PTSD. Soon, the new algorithm should allow doctors to offer targeted prevention to at-risk survivors.
"This study opens a new way to predict post-traumatic disorders, which future work will make more and more accurate," Dr. Arieh Y. Shalev, professor and co-director of NYU's Steven and Alexandra Cohen Veterans Center, told Medical Daily.
What is special about the new algorithm is it includes 800 different variables. There are many moving parts to PTSD, which, unlike many other illnesses, includes a very broad bandwidth of risk factors — including a person’s response to the event, the characteristics of the traumatic event, early symptoms, early physical responses, early neuroendocrine responses, genetics, and circumstance of the recovery environment. Though it is this multitude of potential signs and symptoms that has stymied efforts to create an accurate predictive tool, other problems exist as well.
"Many of the currently known risk indicators may not be present, or not captured in every exposed individual," wrote the authors of the new study. "For example, elevated heart rate response to traumatic events, whilst repeatedly associated with subsequent PTSD is only recorded in survivors who are brought to medical attention." Predicting PTSD is, in a phrase, hugely complicated.
Sophisticated Yet Simple
To develop a new computational tool, Shalev and his co-authors used data collected for the Jerusalem Trauma Outreach and Prevention Study. After screening 4,743 eligible participants — all adults between the ages of 18 and 70 who visited the emergency room following a trauma — Shalev and his team conducted a series of telephone interviews with survivors following their ER visits and, in the case of the 1,502 who showed immediate symptoms of PTSD, additional clinical interviews.
"To get accurate information from interviewees one has to gain their trust and readiness to disclose their experience," Shalev told Medical Daily. "This is particularly important in individuals who have recently experienced a traumatic event." Extensive interviews were necessary, he said, to collect enough accurate information.
Following the data gathering, the researchers devised an algorithm based on the many variables and findings. When applied to data collected within 10 days of a traumatic event, the algorithm accurately predicted those likely to develop PTSD. While the sophisticated tool increases researchers' ability to predict effectively, it includes many very simple and easy to observe factors, such as head trauma, length of stay in the emergency room, and a patient asking for help. The researchers expected and were prepared for some features, such as a brain injury, to influence the development of PTSD, yet other factors startled them.
"Surprisingly, gender was not among the consistent predictors," the researchers noted in their study. In speaking to Medical Daily, Shalev said, "This is surprising because the current literature ... found higher likelihood of PTSD in women." However, a closer look clarified the matter: For the type of trauma studied "even the older literature shows no gender difference," he said.
While the new algorithm was proven effective for this small study, Shalev stresses this is still only a model and it is not yet ready for prime time. The computational tool requires more input from other patient populations to be universally useful. However, the process of gathering that data has already begun. The research team already is collaborating with researchers from Columbia and Harvard who are working to incorporate datasets from 19 other centers worldwide.
Source: Karstoft KI, Galatzer-Levy IR, Statnikov A, Li Z, Shalev AY. Bridging a Translational Gap: Using Machine Learning to Improve the Prediction of PTSD Results from the Jerusalem Trauma Outreach and Prevention Study (J-TOPS). BMC Psychiatry. 2015.