Twitter Can Know When You'll Get Sick Eight Days Before You Do
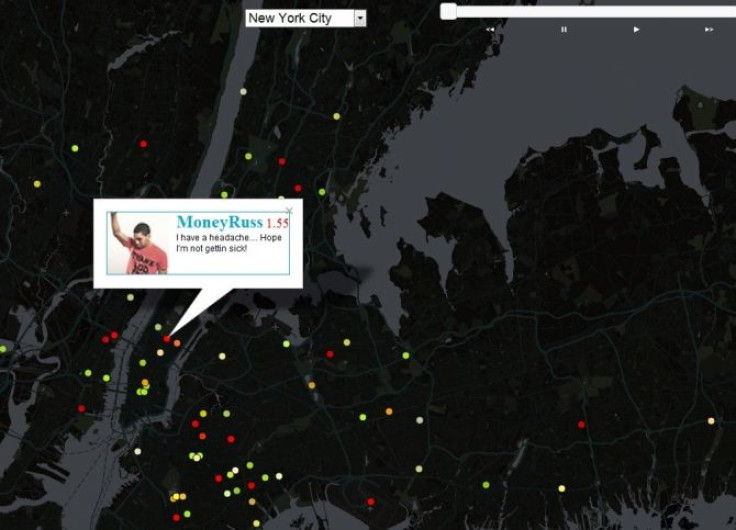
It may sound simple: you walk into a public place, like a subway, a store, or your office, someplace where you will come into contact with a lot of people. Some of those people are sick. A couple days later, you become sick as well.
While it may sound like common sense, a team of researchers has taken those trends and developed them using social media. The University of Rochester's Adam Sadilek, Henry Kautz, and Vincent Silenzio, wanted to know if he could use Twitter to predict flu trends in your area. The paper explains: "Human contact is the single most important factor in the transmission of infectious diseases. Since the contact is often indirect, such as via a doorknob, we focus on a more general notion of colocation."
The idea sounds similar to Google Flu Trends, which analyzes where people are searching for the flu and determines where outbreaks might take place. Sadilek wanted to hone Google Flu Trends' capacity and make it specific for you.
Sadilek and his team analyzed 4.4 million GPS-tagged Tweets from over 600,000 users in New York City over the course of one month in 2010. They trained their machine-learning algorithm to ignore tweets by healthy people – "I am so, so sick of this song!" – and focus instead on actual sick people.
According to the paper, "performance of the CRF is significantly enhanced by including features that are not only based on the health status of friends, but are also based on the estimated encounters with already sick, symptomatic individuals in the dataset, including non-friends. Thus, the model is able to capture the role of locations in the spread of an infectious disease, the impact of the duration of colocation on disease transmission, as well as the delay between a contagion event and the onset of the symptoms."
In other words, their algorithm looked not just at users' friends' health – which, in all likelihood, users would already know – but strangers that they might have encountered.
Researchers could tend determine with a startling amount of accuracy whether healthy people would get sick. Their algorithm was correct 90 percent of the time and about eight days in advance.
The system, of course, has limitations. Not everyone feels the need to update Twitter when they get sick. (Some of us get sick so often that the constant bombardment of updates about our disease history would get us blocked. Cough.) In addition, there are many interlocking factors about what makes a person come down with a disease – while encounters are important, they are not the entire story.
But if nothing else, it might make you that much less suspicious about tagging your GPS location to your Tweets.
You can see Sadilek's work and the real-time health from a handful of cities including New York, Boston, London and Washington D.C. via Corpora.io.
The paper, which received an outstanding mention from the Association for the Advancement of Artificial Intelligence, was presented at the association's conference in Toronto.